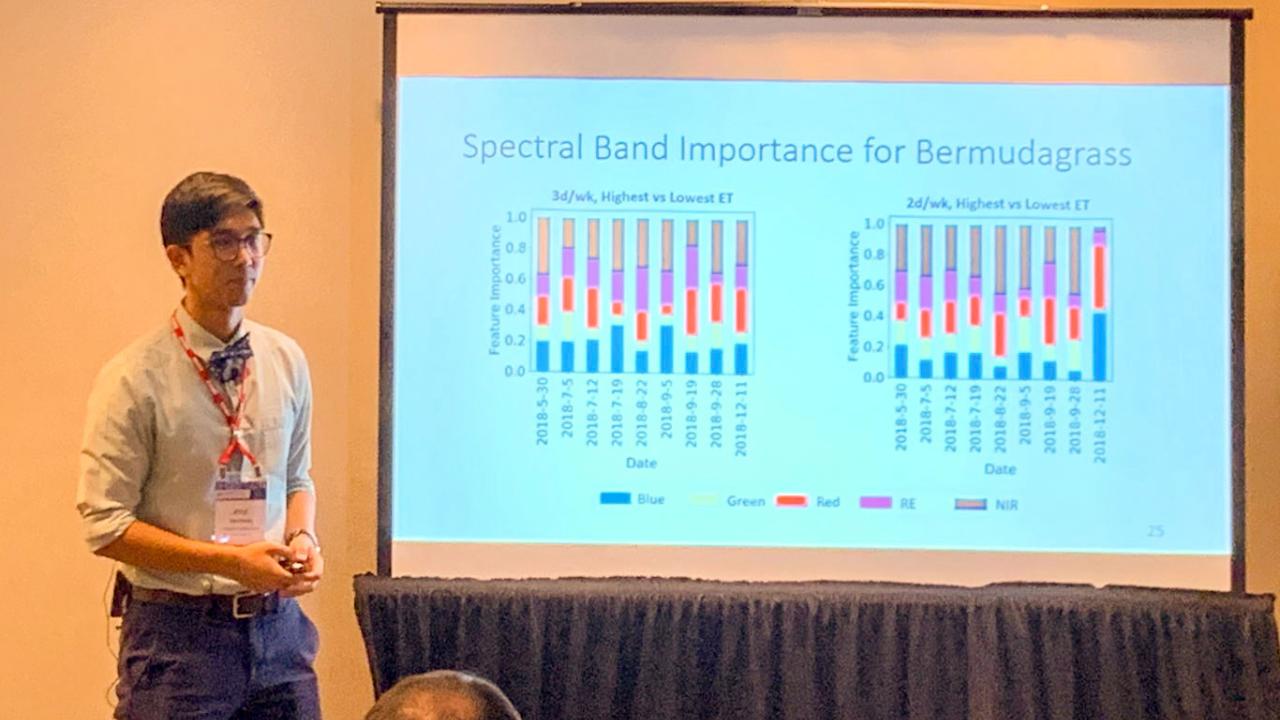
EBS Grad Student Kyle Cheung places in ASABE poster competition
Congratulations to graduate student Kyle Cheung, who placed in the ASABE Oral/Poster Competition within the NRES technical community for his work entitled, “Identification of Drought Stress in Turfgrass Using Multispectral and Hyperspectral Remote Sensing”. Cheung presented this work during the ASABE Annual International Meeting in Boston, Massachusetts in July.
Cheung researches drought stress in Assistant Cooperative Extension Specialist Ali Pourezza's lab.
Read his ASABE AIM 2019 Presentation Abstract:
Title: Identification of Drought Stress in Turfgrass Using Multispectral and Hyperspectral Remote Sensing
Authors:
Kyle Cheung, University of California, Davis; Davis, CA
Alireza Pourreza, University of California, Davis; Davis, CA
German Zuniga Ramirez, University of California, Davis; Parlier, CA
Maggie Reiter, University of California Cooperative Extension; Fresno, CA
Amir Haghverdi, University of California, Riverside; Riverside, CA
Abstract:
Turfgrass, a large component of urban landscapes, has been subject to deficit irrigation as part of water conservation efforts in California. To determine the effectiveness of these irrigation protocols and minimize the drought injury on turfgrass that may be caused, the authors propose a study into a new detection method sensitive to drought stress in turfgrass. Currently, turfgrass quality is evaluated by its visual and texture properties. There have been efforts to correlate multispectral vegetative indices, such as the Normalized Difference Vegetative Index (NDVI) to these human perceived qualities, but do not offer significant improvements over qualitative evaluations. Furthermore, studies that have quantified drought stress through vegetative indices have cautioned that an index can be affected by soil moisture, canopy density, and other vegetative properties. Therefore, an interpretation model customized for drought injury or water stress detection in turfgrass is needed to minimize those effects. The study seeks to take advantage of recent advancements in spectral sensing and machine learning methods to determine the spectral bands that are most sensitive to drought stress in turfgrass. The study also seeks to create a drought index specific for two turfgrass species commonly grown in California, bermudagrass (Cynodon dactylon) and tall fescue (Lolium arundinacea). The study uses data collected by a handheld hyperspectral sensor (200-1650nm) and aerial multispectral camera between 1 May 2018 and 15 December 2018 on field plots established in Fresno, California. Three different deficit irrigation treatments were applied to these field plots; bermudagrass was irrigated at 40, 50, or 60% of reference evapotranspiration (ETo), tall fescue was irrigated at 50, 65, or 80% of ETo. Both the multispectral images and hyperspectral radiometry were used to investigate the two objectives, taking advantage of the spatial and spectral resolution that both datasets offer. The results of this study can be extended as practical recommendations for turfgrass drought stress detection that integrates with existing multispectral sensors.
___________________________________
You can learn more about Cheung's research here.